Discrete Mathematics I - Appendix-B: Algorithm Analysis
Algorithms Manual Analysis (Closures Analysis):
1) N-order looping algorithms:
- Recall, Closure A:
A { FOR I = 1 TO N: command() END }
- Then, it follows that the dummy
command()
with the clock-complexity $N_c$ will be executed $N$ times, so \(f(N) = N * (N_c)\) - Hence, the complexity of the closure execution can be represented as a finite-sum by Riemann’s sum formula: \(f(N) = \sum_{n=1}^{N} N_{c_n} = {(N_c)}_1 + {(N_c)}_2 + {(N_c)}_3 +...+ ({N_c})\_{N-2} + ({N_c})\_{N-1} + ({N_c})\_{N} = N(N_c)\)
- As a matter of multi-variable equations, as well as the total clock-complexity of the execution depends on the number of iterations, it also depends on what’s inside the loop closures, or in other words the
command()
complexity. If, thecommand()
’s clock-complexity can be evaluated to $N_c=1$ as a matter of simple command-execution opcode, then the total clock-complexity for this loop closure is \(f(N) = \sum_{n=1}^{N} 1 = N(1) = N\). - Since, Riemann’s sums can be applied for a finite-set S of closures execution, so \(f(I) = \sum_{i=1}^{I} f(N_{i}) = f(N_{i}) + f(N_{i+1}) + f(N_{i+2}) + ... + f(N_{I-2}) + f(N_{I-1}) + f(N_{I})\)
- Then, a specific notation of Riemann’s sums can be applied for a finite-set $S_L$ of loop closures execution: \(f(I) = \sum_{i=1}^{I} f(L_{i}) = f(L_{i}) + f(L_{i+1}) + f(L_{i+2}) + ... + f(L_{I-2}) + f(L_{I-1}) + f(L_{I})\) \(= L_i + L_{i+1} + L_{i+2} + ... + L_{I-2} + L_{I-1} + L_{I}\) ;where $I$ is the total number of closures, and it represents the index of the finite-item in the set.
2) Conditional closures algorithms:
- Recall, Closure B:
B { IF ({C_i} = {VALUE}) THEN command() END }
- Where, $C_i$ is the condition tag, and i is the number of conditions, in this case, it’s 1 times.
- Then, it follows that the dummy
command()
will be executed $1$ times, so \(f(n) = 1 * N_c\) ;where $N_c$ represents the clock-complexity for the involvedcommand()
to be executed by this execution. - Since, Riemann’s sums can be applied for a finite-set S of closures execution, so \(f(I) = \sum_{i=1}^{I} f(N_{i}) = f(N_{i}) + f(N_{i+1}) + f(N_{i+2}) + ... + f(N_{I-2}) + f(N_{I-1}) + f(N_{I})\)
- Then, a specific notation of Riemann’s sums can be applied for a finite-set $S_C$ of conditional closures execution, so \(f(I) = \sum_{i=1}^{I} f(C_{i}) = f(C_{i}) + f(C_{i+1}) + f(C_{i+2}) + ... + f(C_{I-2}) + f(C_{I-1}) + f(C_{I})\)
\(={(N_c)}\_1 + {(N_c)}\_2 + {(N_c)}\_3 +...+ ({N_c})\_{I-2} + ({N_c})\_{I-1} + ({N_c})\_{I}\) ;where $I$ is the total number of closures, and it represents the index of the finite-item in the set, and $f(C_{i})$ is the complexity of execution of a conditional command
command()
(notice, how this function is very abstract, as thecommand()
could be another algorithm of another complexity, seecompound complexities section
).
3) The scientific basis behind compositing closures (defining a transcendental formula for closures):
-
Closures can be designated as special types of Sets; where operations, a specific sort of relations, are being monitored in an execution environment, hence all types of closures creates \(f(N) = C(N) * \sum_{c=1}^{C} N_c = C(N) * (N_1 + N_2 + N_3 + ... + N_{C-2} + N_{C-1} + N_C)\) ;where $f(N)$ is the total clock-complexity of the closure execution (execution of commands inside the closure), $C(N)$ is the clock-complexity of the closure itself by its class (e.g., first-order loops use $C(N)=N$), and $N_c$ represents the clock-complexity of the single command, in which their Riemann’s sum yields the total clock-complexity of execution of the enclosed commands.
-
It follows that this could be also represented using the integral function, aka. Leibniz’s notation, the integral function integrates the time complexities of the stack of the function in the form $f(x).dx=C(N).N_c$: \(Since, f(x) = F(x) = \int_a^x{f'(x).dx}\) \(Hence, F(x_1) - F(x_0) = \int_a^{x_1}{f'(x).dx} - \int_a^{x_0}{f'(x).dx} = \int_{x_0}^{x_1}{f'(x).dx} = f(x_1) - f(x_0)\) \(Then, F(x) = f(N) = C(N) * \sum_{c=1}^{C} N_c = \sum_{c=1}^{C} N_c * C(N) = \int_1^C{f'(x).dx} = f(C) - f(1)\)
-
Almost all properties of Sets could be applied to closures, hence if the super-closure (superset) has a simple complexity of constant functional execution (i.e., of $C(N) = 1$), then the generalized Riemann’s sum can be narrowed down to: \(f(N) = C(N) * \sum_{c=1}^{C} N_c = (1) * \sum_{c=1}^{C} N_c\)
-
While, if the super-closure (superset) has a loop complexity of transcendental functional execution (i.e., of $C(N) = c*N^e$), then the generalized Riemann’s sum can be obtained as follows: \(f(N) = C(N) * \sum_{c=1}^{C} N_c = c * N^e * \sum_{c=1}^{C} N_c\) ;where $c$ is a constant co-efficient, and $e$ is the exponent representing nested loop closures.
4) Compound (or Nested) closures algorithms:
- Recall, a super-closure $S_c$; such that:
S_c: { command() }
- Then, it follows that this closure executes the
command()
in (N) times the clock-complexity of the command $N_c$, hence \(f(N) = N*N_c\) - Hence, if the
command()
holds the following closure as its stack:command(): { FOR I = 1 TO N: command1(); END }
- Then, the total clock-complexity of execution will be: \(f(N) = N*N_c\)
- However, if the
command()
holds a simple closure as the follows:command(): { IF ({C_i} = {VALUE}) THEN command1() END }
- Then, it follows that the total clock-complexity of execution will be: \(f(N) = N*N_c = (1) * N_c = N_c\)
- Now, if the
command()
holds a nested loop closure as follows:command(): { FOR I = 1 TO N: FOR J = 1 TO N: command1(); END END }
-
Then, it follows that the total clock-complexity can be evaluated to: \(f(N) = N*N_c = N * (N * N_c') = N^2 * N_c'\) ;which means that the
command1()
where $N_c’$ will be executed $N^2$ times, in a product set fashion. - Now, the $N_c$ can represent any type of clock-complexity ranging from complexity to compund complexity involving finite-sets, the general formula utilizes Riemann’s sum, and can be also represented as an integral function using Leibniz’s notation.
Our Features
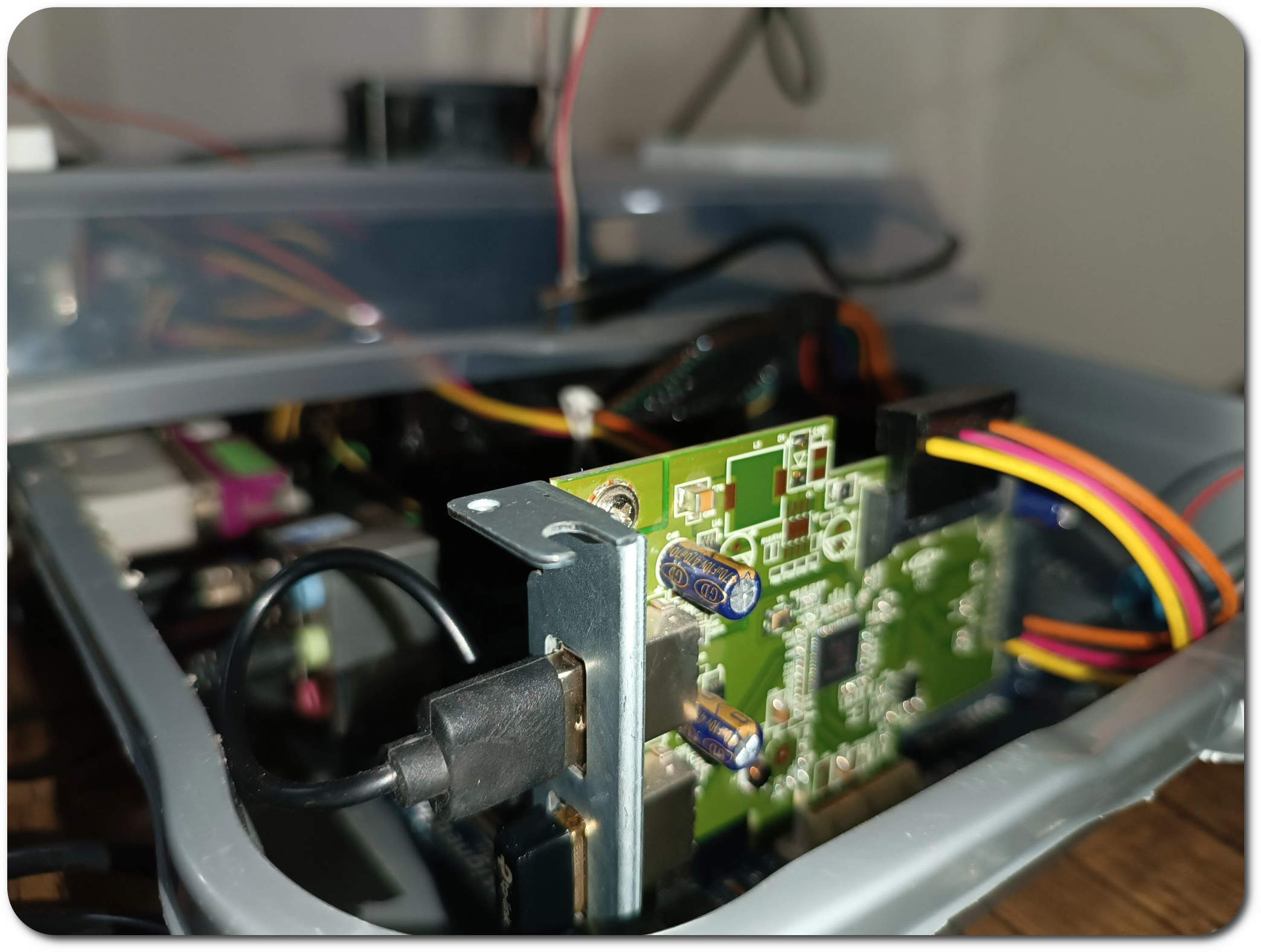
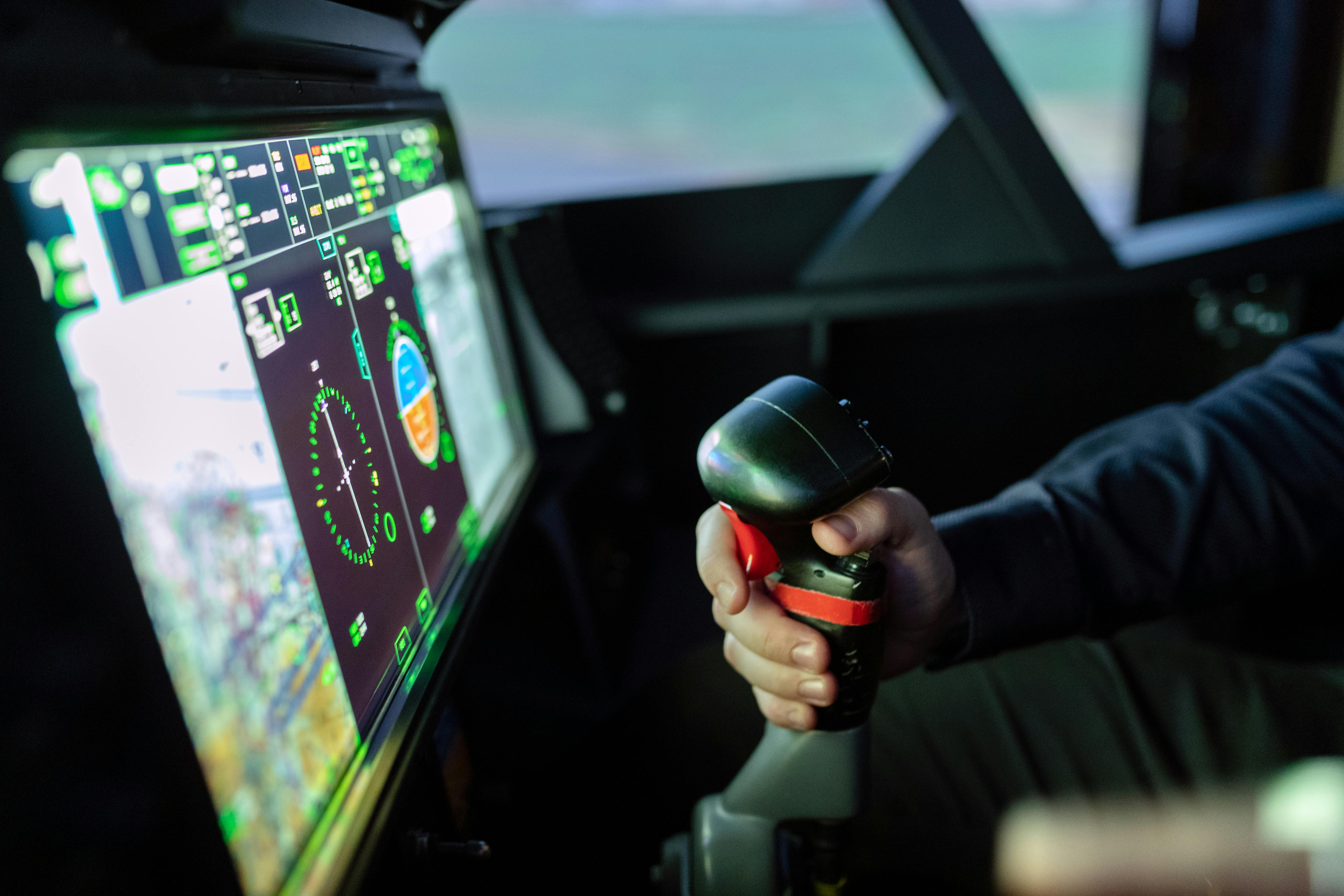
Distributed Simulation
An overview of distributed simulation systems.
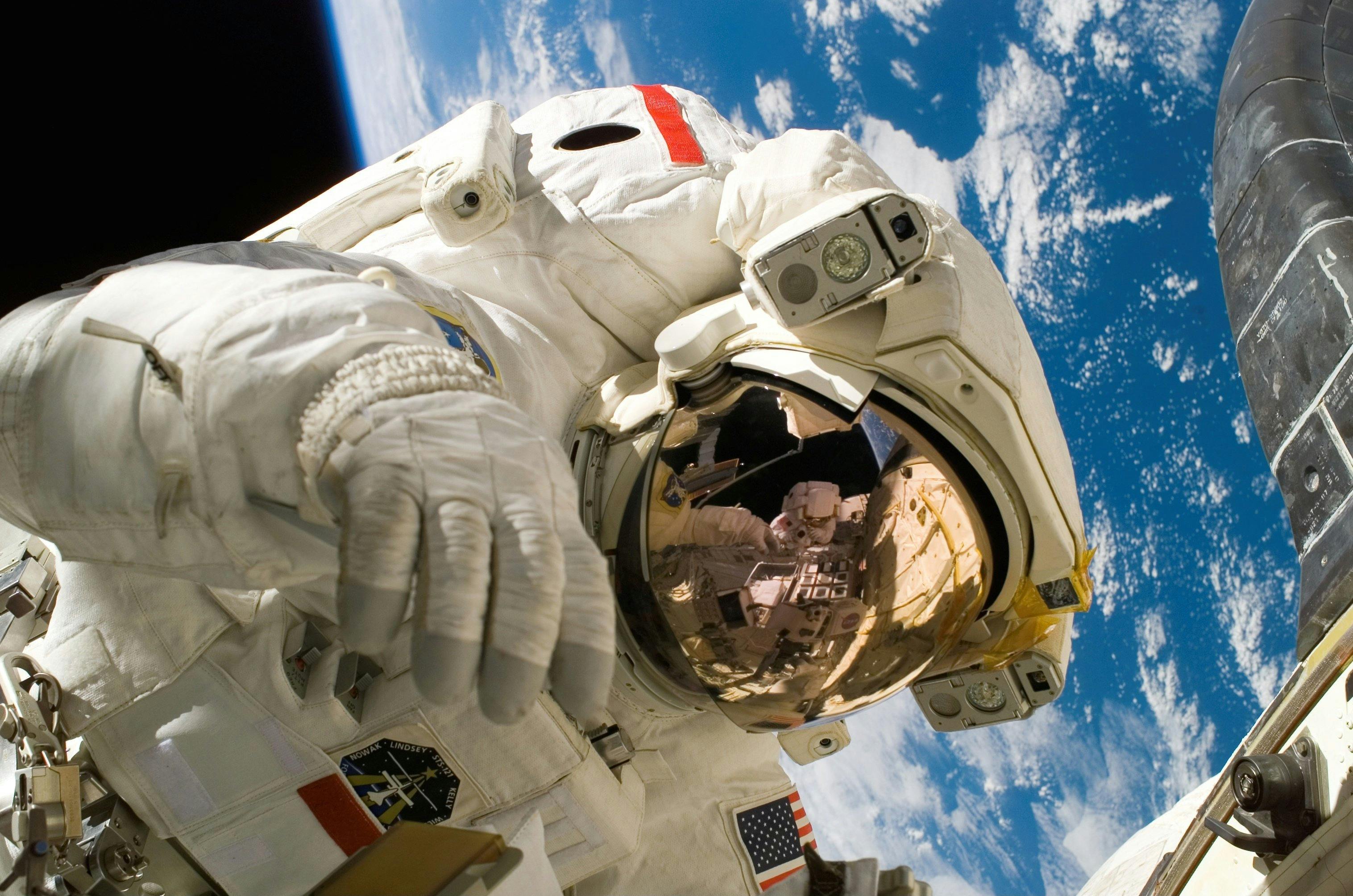
NASA DSES Project
Insights into the Distributed Space Exploration Simulation System project of NASA.
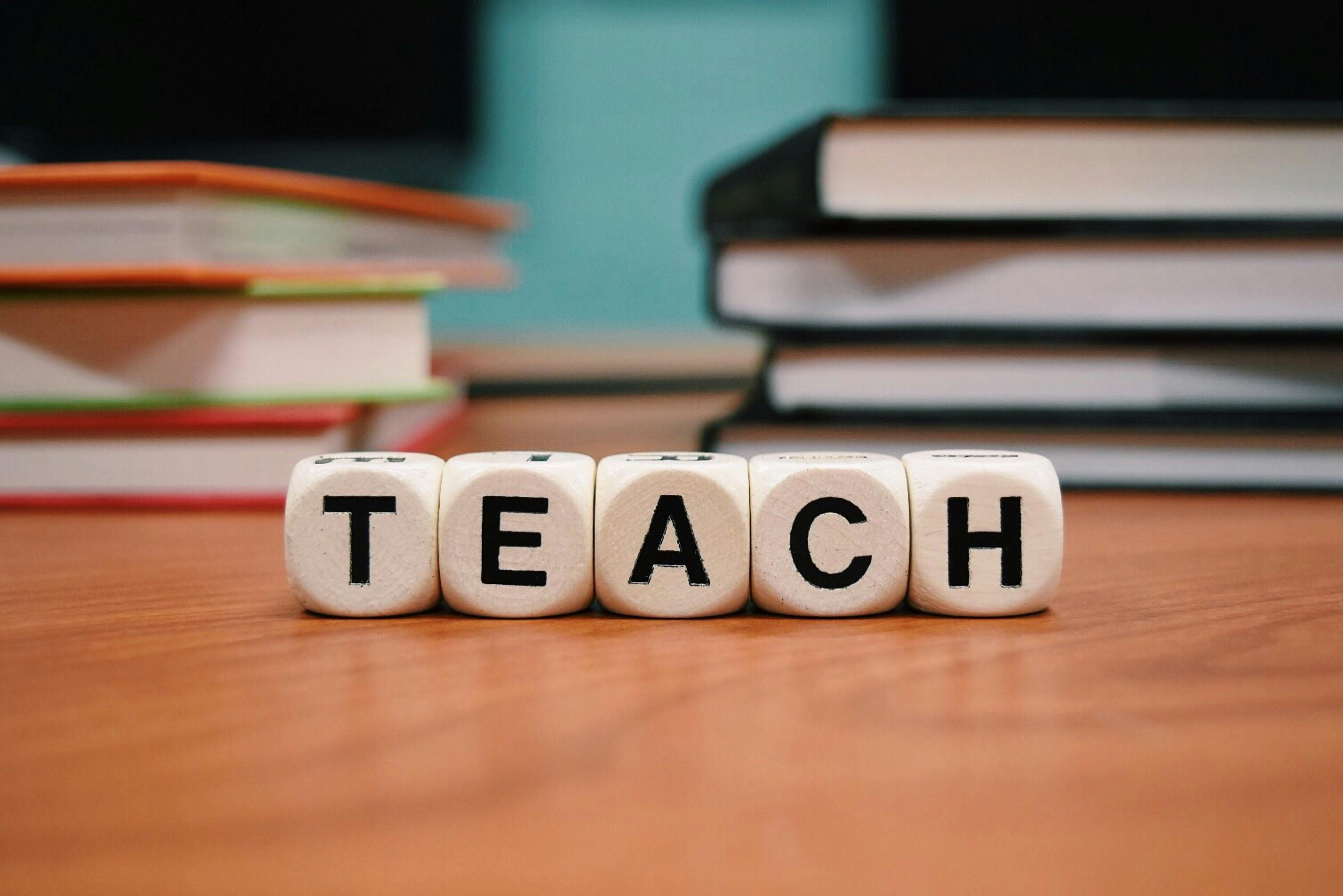
Educational Applications
How educational institutions can benefit from simulation systems.
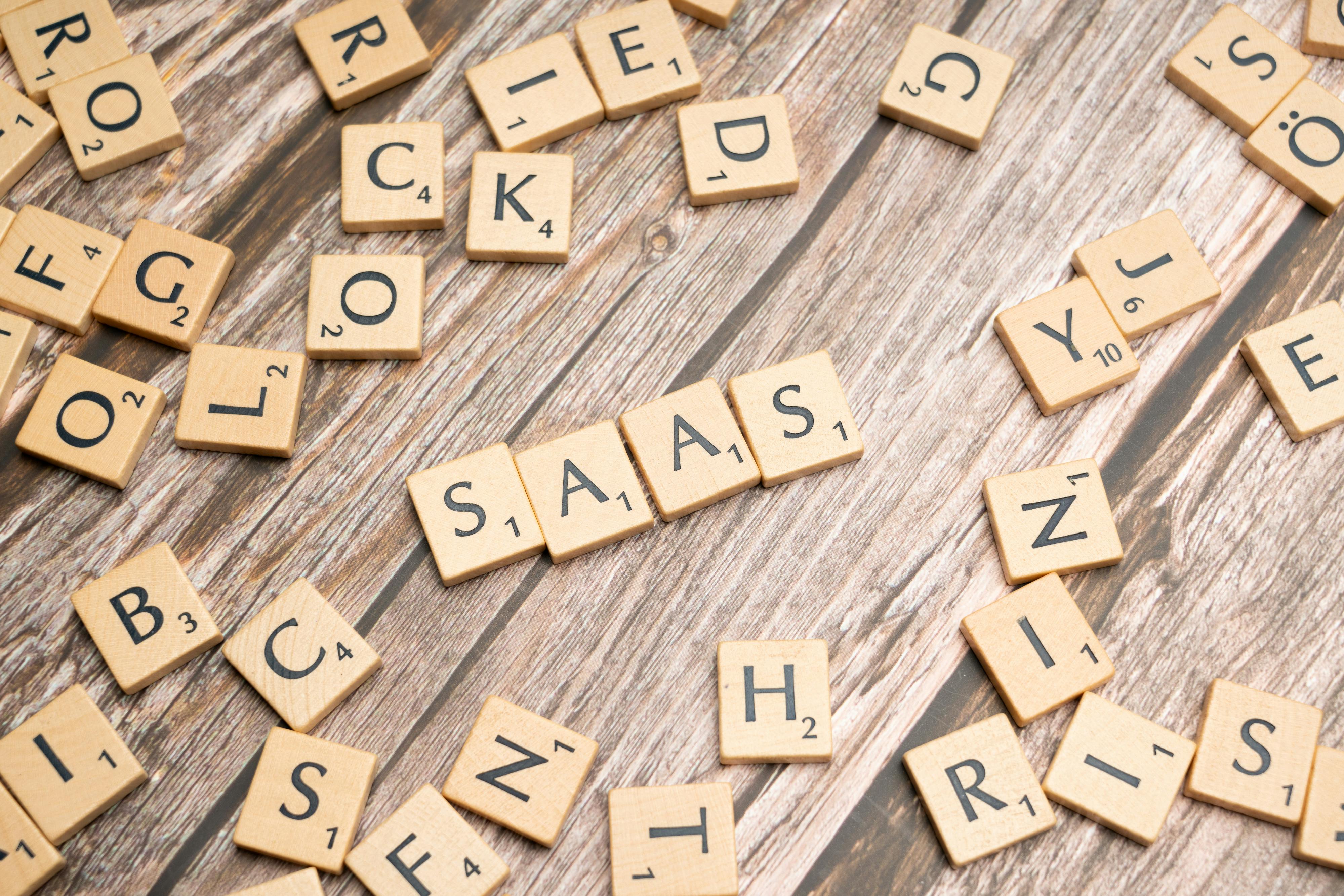
Scalable Solutions
Implementing scalable solutions for various needs.
Contacts:
Name
Pavly Gerges
pepogerges33@gmail.com
Tel
Address
Egypt, Cairo